arge language models, or artificial intelligence (AI) models trained on large swaths of text, could one day expedite online disinformation research, according to a new study from DisinfoLab, a student-led research lab at the College of William & Mary’s Global Research Institute.
In June of 2020, OpenAI made headlines for releasing its new large language model, GPT-3. This model was lauded for its ability to produce human-like text and perform a variety of natural language processing tasks with ease. Since GPT-3’s release, other technology companies including Google, Meta, Deepmind, and Microsoft have continued to push the bounds of AI with their own large language models. These models have immense potential, from revolutionizing the way we search the web to translating natural language into code.
Although the prospects of large language models are exciting, these tools are also a cause for concern. Bias in AI is well documented, and large language models are no exception. A previous study by DisinfoLab, published in January of 2022, found that GPT-3-generated text completions exhibited high rates of bias concerning gender; sexual orientation and sexuality; race, ethnicity and nationality; and religion. A follow-up study on InstructGPT—OpenAI’s newest language model marketed as less biased and toxic—found that little had changed outside of a decrease in rates of religious bias. These biased language models will help spread stereotypes and disinformation, especially as companies begin to power consumer-facing tools like search engines with this AI.
Manual Comment Analysis
Still, skillful deployment of large language models could one day facilitate quicker and easier disinformation research by automating qualitative analysis. Consider DisinfoLab’s latest study on the vulnerability of various Eastern European populations to disinformation.
Analysts collected 110 Facebook posts promoting false information and 820 Hungarian, Polish, and Russian language comments replying to them. Next, analysts manually reviewed each comment and, using a set of criteria, categorized the comment as either agreeing with, disagreeing with, or taking no clear position on the disinformation contained in the corresponding post. To ensure rigorous categorization, two analysts independently categorized each comment and reached consensus on any disparate categorizations.
Manual Analysis vs. InstructGPT Analysis
Qualitative comment coding is time-intensive, labor-intensive, and repetitive. But with large language models, we may one day automate this step of the research process. To gauge the current capacity of AI to conduct this qualitative analysis, DisinfoLab replicated their analysis of the same set of Facebook comments, swapping out human analysts for InstructGPT. Specifically, analysts presented InstructGPT with a Facebook post containing disinformation and one of its comments. The analysts prompted InstructGPT to determine whether the comment agreed with, disagreed with, or neither agreed/disagreed with the content of the original post.
The results below are the result of repeated experimentation with InstructGPT settings, prompt phrasings, and even training with subsets of the manually categorized data set, to achieve the most accurate results.
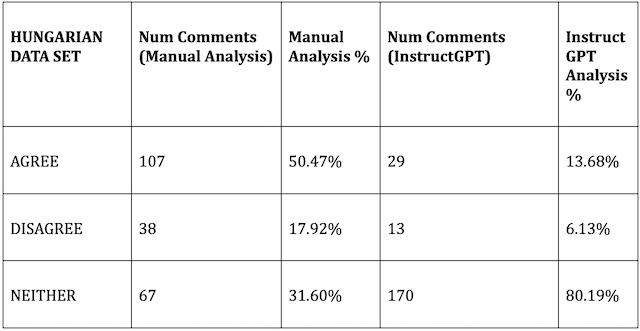

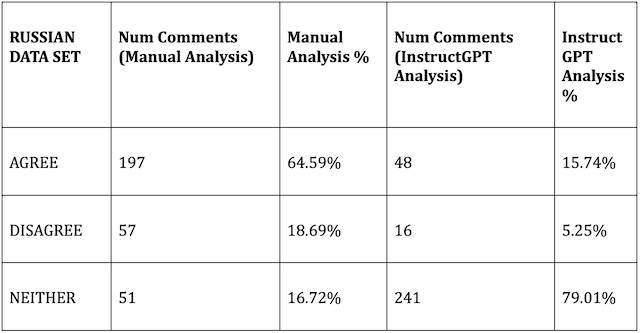
There was a stark difference between human judgment and InstructGPT analysis for all three languages. DisinfoLab analysts and InstructGPT categorized only 57 out of 212 comments in the Hungarian data set identically, or 26.89% of comments in the set. For the Polish data set, only 71 out of 303 comments, or 23.43% of comments in the set were coded identically. And for the Russian data set, only 67 out of 305 comments, or 21.97% of comments in the set were coded identically.
When considering only the comments that InstructGPT categorized as either agree or disagree, its accuracy rate dramatically improved. Of such comments, 45.24% of Hungarian comments, 52.38% of Polish comments, and 46.97% of Russian comments were coded identically by DisinfoLab analysts and InstructGPT.
Viability of Large Language Model-Powered Analysis
In DisinfoLab’s comparison of manual versus AI qualitative analysis, InstructGPT failed to offer a viable alternative methodology for the Eastern Europe study. However, DisinfoLab found two practical conclusions that may aid future research.
First, across all three languages, InstructGPT heavily erred toward categorizing comments as neither agreeing nor disagreeing with corresponding posts. Interestingly, the ratio of number of agreement to disagreement comments found by both DisinfoLab analysts and InstructGPT are comparable.
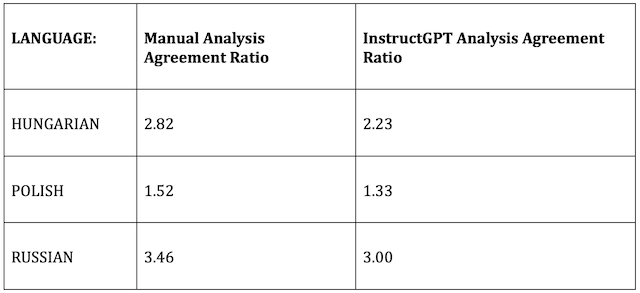
This finding suggests that although InstructGPT analysis may be poor at judging individual comments, the model can still be effective at determining the overall agreement/disagreement sentiment of a group of commenters.
Second, InstructGPT’s tendency to mark comments as “neither” is likely due to the model’s limited comprehension of the subject matter at hand. While posts in the study primarily focused on the ongoing war in Ukraine and COVID-19, InstructGPT’s training data only contained content through October 2019. If InstructGPT were to perform a comparable task for comments on topics with substantial training data, it may perform differently.
Implications
Understanding both the limitations and the potential for large language models in conducting analysis is critical to the disinformation research field. Larger sets of social media data allow for more rigorous research and a better understanding into the dynamics of online disinformation. But the resource-intensive nature of qualitative analysis limits researcher’s capacity to engage with such sets in their entirety.
Analyzing ~800 comments for the Eastern Europe study took six analysts approximately one week to complete. Performing analyses with much larger datasets - as will likely be necessary as we scale up efforts to combat disinformation - is infeasible with fully manual analysis. But it can be done with a hybrid approach, wherein AI takes on the bulk of the work and humans manually audit samples of the resulting data. Such an approach would allow researchers to scale high quality analysis to sizable datasets.
Researchers have long urged tech companies to recognize the potential dangers of large language models and take appropriate steps to mitigate them. But tech companies can also proactively help combat disinformation by investing in their models’ ability to conduct text-focused qualitative analysis. Packaging these capabilities into a turnkey service could change the game for disinformation research.
Fortunately, large language model development is a prolific field. On July 11th, 2022, Meta AI announced the release of “Sphere,” an open-source repository of millions of documents and text from throughout the web. Given large-language model’s necessity for huge sets of training data, Sphere may open the space to new developers, and offer more training ground for existing models. DisinfoLab urges these actors to invest in the positive potential of AI to aid research.
a global affairs media network
Large Language Model AI Can Lead Efforts to Combat Disinformation
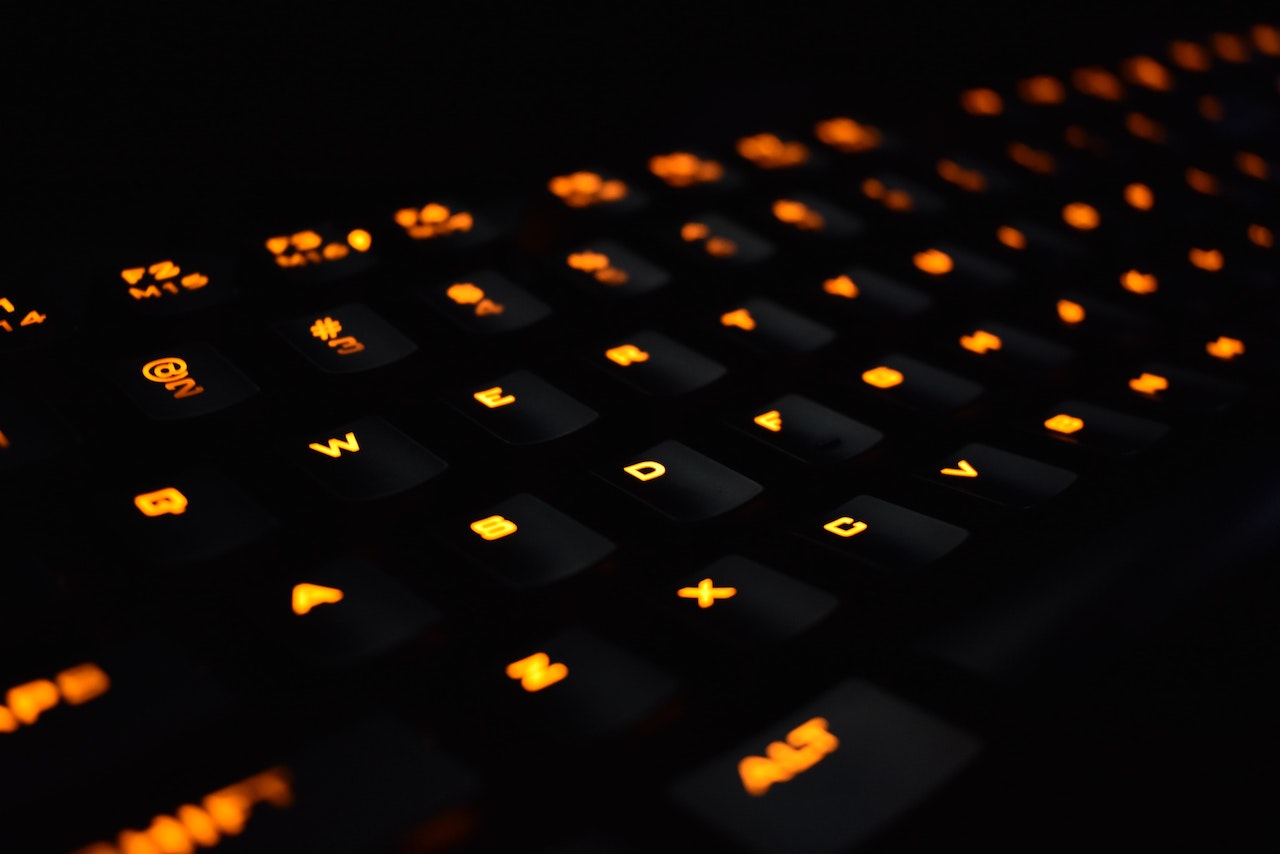
Photo by blurrystock via Unsplash.
August 19, 2022
Large language models, or artificial intelligence (AI) models trained on large swaths of text, could one day expedite online disinformation research, say DisinfoLab's Jeremy Swack, Aaraj Vij, and Thomas Plant.
L
arge language models, or artificial intelligence (AI) models trained on large swaths of text, could one day expedite online disinformation research, according to a new study from DisinfoLab, a student-led research lab at the College of William & Mary’s Global Research Institute.
In June of 2020, OpenAI made headlines for releasing its new large language model, GPT-3. This model was lauded for its ability to produce human-like text and perform a variety of natural language processing tasks with ease. Since GPT-3’s release, other technology companies including Google, Meta, Deepmind, and Microsoft have continued to push the bounds of AI with their own large language models. These models have immense potential, from revolutionizing the way we search the web to translating natural language into code.
Although the prospects of large language models are exciting, these tools are also a cause for concern. Bias in AI is well documented, and large language models are no exception. A previous study by DisinfoLab, published in January of 2022, found that GPT-3-generated text completions exhibited high rates of bias concerning gender; sexual orientation and sexuality; race, ethnicity and nationality; and religion. A follow-up study on InstructGPT—OpenAI’s newest language model marketed as less biased and toxic—found that little had changed outside of a decrease in rates of religious bias. These biased language models will help spread stereotypes and disinformation, especially as companies begin to power consumer-facing tools like search engines with this AI.
Manual Comment Analysis
Still, skillful deployment of large language models could one day facilitate quicker and easier disinformation research by automating qualitative analysis. Consider DisinfoLab’s latest study on the vulnerability of various Eastern European populations to disinformation.
Analysts collected 110 Facebook posts promoting false information and 820 Hungarian, Polish, and Russian language comments replying to them. Next, analysts manually reviewed each comment and, using a set of criteria, categorized the comment as either agreeing with, disagreeing with, or taking no clear position on the disinformation contained in the corresponding post. To ensure rigorous categorization, two analysts independently categorized each comment and reached consensus on any disparate categorizations.
Manual Analysis vs. InstructGPT Analysis
Qualitative comment coding is time-intensive, labor-intensive, and repetitive. But with large language models, we may one day automate this step of the research process. To gauge the current capacity of AI to conduct this qualitative analysis, DisinfoLab replicated their analysis of the same set of Facebook comments, swapping out human analysts for InstructGPT. Specifically, analysts presented InstructGPT with a Facebook post containing disinformation and one of its comments. The analysts prompted InstructGPT to determine whether the comment agreed with, disagreed with, or neither agreed/disagreed with the content of the original post.
The results below are the result of repeated experimentation with InstructGPT settings, prompt phrasings, and even training with subsets of the manually categorized data set, to achieve the most accurate results.
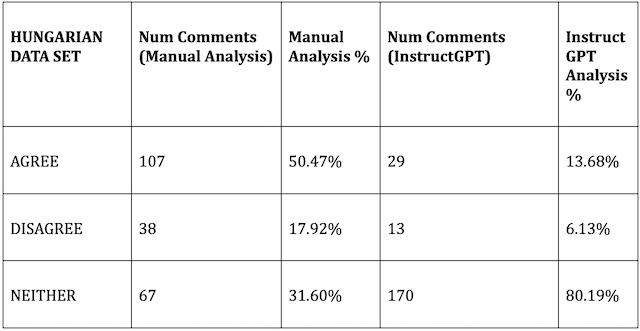

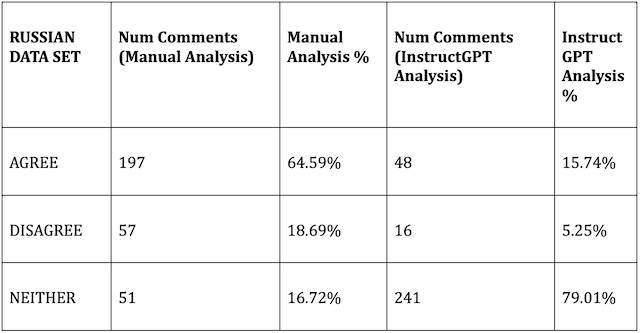
There was a stark difference between human judgment and InstructGPT analysis for all three languages. DisinfoLab analysts and InstructGPT categorized only 57 out of 212 comments in the Hungarian data set identically, or 26.89% of comments in the set. For the Polish data set, only 71 out of 303 comments, or 23.43% of comments in the set were coded identically. And for the Russian data set, only 67 out of 305 comments, or 21.97% of comments in the set were coded identically.
When considering only the comments that InstructGPT categorized as either agree or disagree, its accuracy rate dramatically improved. Of such comments, 45.24% of Hungarian comments, 52.38% of Polish comments, and 46.97% of Russian comments were coded identically by DisinfoLab analysts and InstructGPT.
Viability of Large Language Model-Powered Analysis
In DisinfoLab’s comparison of manual versus AI qualitative analysis, InstructGPT failed to offer a viable alternative methodology for the Eastern Europe study. However, DisinfoLab found two practical conclusions that may aid future research.
First, across all three languages, InstructGPT heavily erred toward categorizing comments as neither agreeing nor disagreeing with corresponding posts. Interestingly, the ratio of number of agreement to disagreement comments found by both DisinfoLab analysts and InstructGPT are comparable.
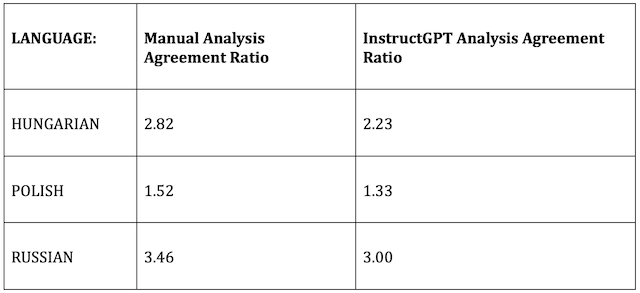
This finding suggests that although InstructGPT analysis may be poor at judging individual comments, the model can still be effective at determining the overall agreement/disagreement sentiment of a group of commenters.
Second, InstructGPT’s tendency to mark comments as “neither” is likely due to the model’s limited comprehension of the subject matter at hand. While posts in the study primarily focused on the ongoing war in Ukraine and COVID-19, InstructGPT’s training data only contained content through October 2019. If InstructGPT were to perform a comparable task for comments on topics with substantial training data, it may perform differently.
Implications
Understanding both the limitations and the potential for large language models in conducting analysis is critical to the disinformation research field. Larger sets of social media data allow for more rigorous research and a better understanding into the dynamics of online disinformation. But the resource-intensive nature of qualitative analysis limits researcher’s capacity to engage with such sets in their entirety.
Analyzing ~800 comments for the Eastern Europe study took six analysts approximately one week to complete. Performing analyses with much larger datasets - as will likely be necessary as we scale up efforts to combat disinformation - is infeasible with fully manual analysis. But it can be done with a hybrid approach, wherein AI takes on the bulk of the work and humans manually audit samples of the resulting data. Such an approach would allow researchers to scale high quality analysis to sizable datasets.
Researchers have long urged tech companies to recognize the potential dangers of large language models and take appropriate steps to mitigate them. But tech companies can also proactively help combat disinformation by investing in their models’ ability to conduct text-focused qualitative analysis. Packaging these capabilities into a turnkey service could change the game for disinformation research.
Fortunately, large language model development is a prolific field. On July 11th, 2022, Meta AI announced the release of “Sphere,” an open-source repository of millions of documents and text from throughout the web. Given large-language model’s necessity for huge sets of training data, Sphere may open the space to new developers, and offer more training ground for existing models. DisinfoLab urges these actors to invest in the positive potential of AI to aid research.