year ago, I sat at a table in one of America’s 2400 job centers watching a job coach help a recently unemployed client navigate their pathway back to work. The coach asked about prior experience and skill sets, whether the client wanted to find a similar job or make a major career change, and what additional supports they would need at this critical time of transition. The coach gave them personalized guidance, connections, and lots of helpful resources. They left the building with more hope in their eyes than when they entered.
A week ago, I sat on a Zoom call watching a similar scene, only this time with a digital makeover courtesy of COVID-19. Many of the same questions were asked and answered, and the job seeker received tailored advice and links to the programs and resources they needed. There was one big difference: in this career navigation session, it wasn’t a job coach giving the advice, it was a chatbot.
The future of education, work, and career navigation is algorithmic. Algorithms, data, and AI are changing the face of how we learn and work. We can all expect our personal and professional journeys to be guided by AI algorithms giving us recommendations on which online course to take, which jobs to apply for, and which candidates to interview and hire. This transition was well underway before the global pandemic hit. COVID-19 just moved up the launch date.
The Good, the Bad, and the Biased
As a data scientist, this algorithmic future is at once thrilling and terrifying to me.
It’s thrilling because the responsible use of algorithms, data, and AI can help a lot of folks in need. AI algorithms could provide personalized career recommendations and instruction to millions of unemployed workers simultaneously without breaking a sweat. With unemployment in the U.S. higher than at any point since the Great Depression, it would be irresponsible not to invest in AI aimed at helping reskill and rehire millions or workers. The need for beneficial and assistive AI in education, career navigation, and hiring will only grow over time as automation continues to disrupt the labor market. We need the same AI tools that put us out of work to help us get back to work with new skills.
But the algorithmic future of education and work also terrifies me--and not because I’m worried about a pending robot uprising or automation taking all our jobs tomorrow. The potential negative impacts of AI on society are much closer, much less apocalyptic, and much more pernicious.
AI does one thing extraordinarily well: learn how to repeat patterns from the past. We can feed mountains of data about hiring decisions to an algorithm, with every human foible and systemic bias perfectly catalogued, and the algorithm will be able to recreate those same biases in hiring with remarkable precision, speed, and scale. This is the algorithmic future of work that tech platforms and startups are racing toward, while failing to acknowledge one incredibly inconvenient truth: as a society we have yet to do the slow, difficult work necessary to ensure the algorithms we deploy tomorrow won’t amplify the systemic inequities and biases of today.
How to Build an Anti-racist Algorithm
We have to do better than the past. The tragic deaths of George Floyd, Breonna Taylor, and too many others have finally forced a long-overdue national reckoning with systemic racism and inequity. Communities are collectively reexamining the roles of statues, textbooks, team names, and entire institutions in carrying the inequities of the past forward into the present. But systemic racism, bias, and inequity are embedded in our data as well. For the future of work to look different than the past, this means examining our databases of job descriptions, hiring decisions, credentials earned, and skills acquired to root out present bias before we build our algorithms. If we don’t, these inequities will be subtly enshrined in the algorithms carrying us into the future.
The good news is, we don’t have to throw out the algorithmic baby with the digital bathwater. We have a playbook for doing better. Whether you are researching, funding, building, or buying AI algorithms for education, career navigation, or work, here are six essential steps you can take to contribute to responsible AI for the future of work:
• Build a diverse coalition of committed stakeholders who will advise and direct collaborative AI design.
• Center on equity as a goal that you will all work toward and measure against.
• Design for the learner or worker first and guarantee genuine digital ownership and empowerment.
• Develop inclusive governance over the data and the algorithms that can use it.
• Ensure transparency and explainability of your algorithms and their impacts.
• Hold yourself and others accountable for missteps and failures and fix them.
Digital Justice in the Future of Work
The potential benefits from AI in education and work are too massive to stop, and the potential harms too consequential to ignore. The work ahead is to bend the arc of innovation toward social equity and individual empowerment. Data should move at the speed of trust. Algorithms should be deployed at the pace of justice. This isn’t too much to ask. It’s the right thing to do. There are coalitions and alliances committed to equitable digital transformation doing this work right now. You can join. They include the T3 Innovation Network, the Open Skills Stack Alliance, the Partnership on AI, the Trust Over IP Network, and many others. Yes, the future of education and work has an equity problem, but if more of us come to the table and lift up our own and others’ voices on this issue, I’m hopeful we can turn the tide.
a global affairs media network
The Revolution Hasn’t Been Digitized. It Needs to Be.
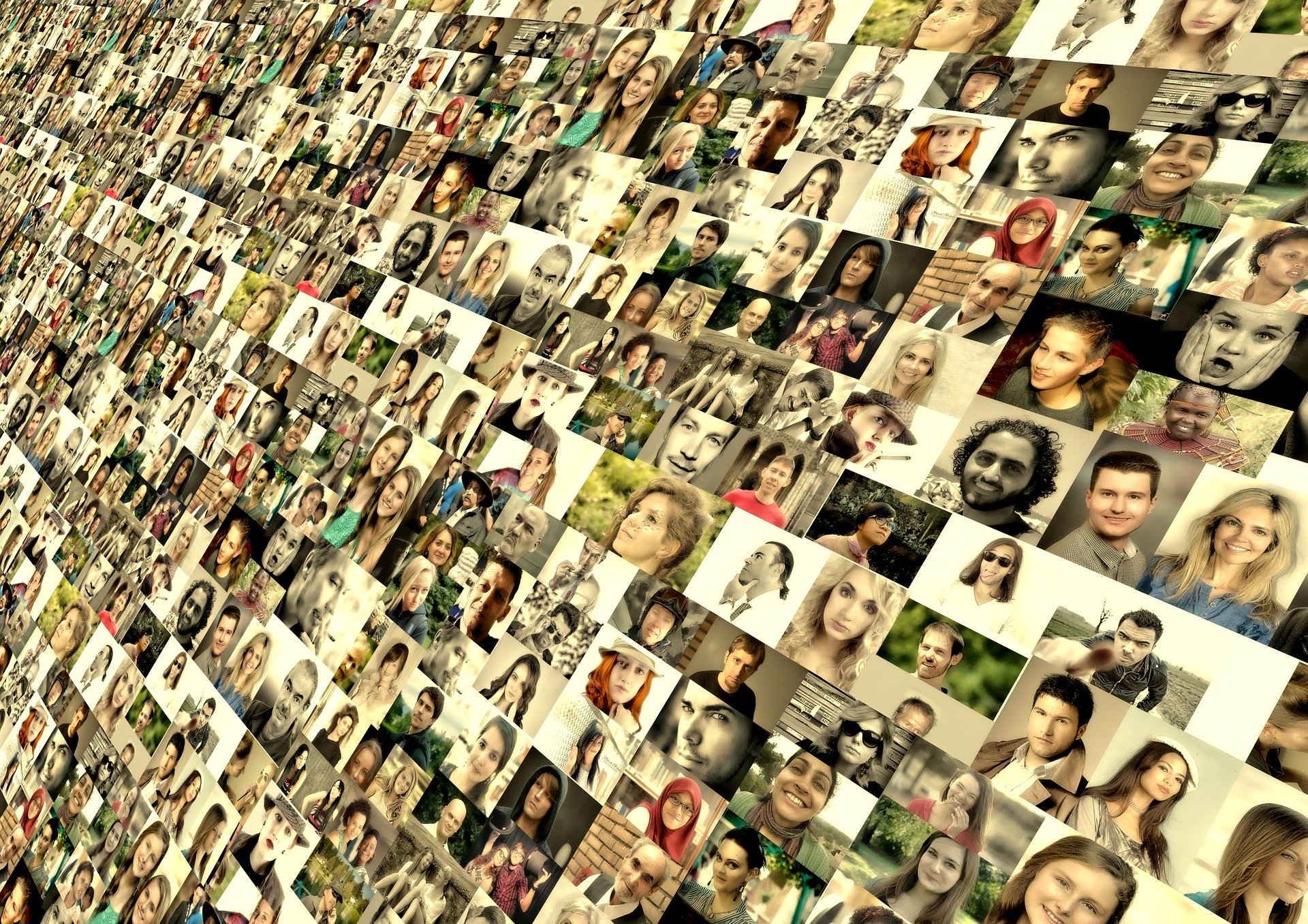
August 3, 2020
The future of education and work has a hidden equity problem, and it’s on all of us to fix it.
A
year ago, I sat at a table in one of America’s 2400 job centers watching a job coach help a recently unemployed client navigate their pathway back to work. The coach asked about prior experience and skill sets, whether the client wanted to find a similar job or make a major career change, and what additional supports they would need at this critical time of transition. The coach gave them personalized guidance, connections, and lots of helpful resources. They left the building with more hope in their eyes than when they entered.
A week ago, I sat on a Zoom call watching a similar scene, only this time with a digital makeover courtesy of COVID-19. Many of the same questions were asked and answered, and the job seeker received tailored advice and links to the programs and resources they needed. There was one big difference: in this career navigation session, it wasn’t a job coach giving the advice, it was a chatbot.
The future of education, work, and career navigation is algorithmic. Algorithms, data, and AI are changing the face of how we learn and work. We can all expect our personal and professional journeys to be guided by AI algorithms giving us recommendations on which online course to take, which jobs to apply for, and which candidates to interview and hire. This transition was well underway before the global pandemic hit. COVID-19 just moved up the launch date.
The Good, the Bad, and the Biased
As a data scientist, this algorithmic future is at once thrilling and terrifying to me.
It’s thrilling because the responsible use of algorithms, data, and AI can help a lot of folks in need. AI algorithms could provide personalized career recommendations and instruction to millions of unemployed workers simultaneously without breaking a sweat. With unemployment in the U.S. higher than at any point since the Great Depression, it would be irresponsible not to invest in AI aimed at helping reskill and rehire millions or workers. The need for beneficial and assistive AI in education, career navigation, and hiring will only grow over time as automation continues to disrupt the labor market. We need the same AI tools that put us out of work to help us get back to work with new skills.
But the algorithmic future of education and work also terrifies me--and not because I’m worried about a pending robot uprising or automation taking all our jobs tomorrow. The potential negative impacts of AI on society are much closer, much less apocalyptic, and much more pernicious.
AI does one thing extraordinarily well: learn how to repeat patterns from the past. We can feed mountains of data about hiring decisions to an algorithm, with every human foible and systemic bias perfectly catalogued, and the algorithm will be able to recreate those same biases in hiring with remarkable precision, speed, and scale. This is the algorithmic future of work that tech platforms and startups are racing toward, while failing to acknowledge one incredibly inconvenient truth: as a society we have yet to do the slow, difficult work necessary to ensure the algorithms we deploy tomorrow won’t amplify the systemic inequities and biases of today.
How to Build an Anti-racist Algorithm
We have to do better than the past. The tragic deaths of George Floyd, Breonna Taylor, and too many others have finally forced a long-overdue national reckoning with systemic racism and inequity. Communities are collectively reexamining the roles of statues, textbooks, team names, and entire institutions in carrying the inequities of the past forward into the present. But systemic racism, bias, and inequity are embedded in our data as well. For the future of work to look different than the past, this means examining our databases of job descriptions, hiring decisions, credentials earned, and skills acquired to root out present bias before we build our algorithms. If we don’t, these inequities will be subtly enshrined in the algorithms carrying us into the future.
The good news is, we don’t have to throw out the algorithmic baby with the digital bathwater. We have a playbook for doing better. Whether you are researching, funding, building, or buying AI algorithms for education, career navigation, or work, here are six essential steps you can take to contribute to responsible AI for the future of work:
• Build a diverse coalition of committed stakeholders who will advise and direct collaborative AI design.
• Center on equity as a goal that you will all work toward and measure against.
• Design for the learner or worker first and guarantee genuine digital ownership and empowerment.
• Develop inclusive governance over the data and the algorithms that can use it.
• Ensure transparency and explainability of your algorithms and their impacts.
• Hold yourself and others accountable for missteps and failures and fix them.
Digital Justice in the Future of Work
The potential benefits from AI in education and work are too massive to stop, and the potential harms too consequential to ignore. The work ahead is to bend the arc of innovation toward social equity and individual empowerment. Data should move at the speed of trust. Algorithms should be deployed at the pace of justice. This isn’t too much to ask. It’s the right thing to do. There are coalitions and alliances committed to equitable digital transformation doing this work right now. You can join. They include the T3 Innovation Network, the Open Skills Stack Alliance, the Partnership on AI, the Trust Over IP Network, and many others. Yes, the future of education and work has an equity problem, but if more of us come to the table and lift up our own and others’ voices on this issue, I’m hopeful we can turn the tide.