n 2009, in the midst of the global financial crisis, Paul Volcker, the former Federal Reserve chair, famously observed that the only socially productive financial innovation of the preceding 20 years was the automated teller machine. One wonders what Volcker would make of the tsunami of digitally enabled financial innovations today, from mobile payment platforms to internet banking and peer-to-peer lending.
Volcker might be reassured: like the humble ATM, many of these innovations have tangible benefits in terms of lowering transactions costs. But as a critic of big financial firms, Volcker presumably also would worry about the entry of some very large technology companies into the sector. Their names are as familiar as their services are ubiquitous: e-commerce behemoth Amazon in the United States, messaging company Kakao in Korea, on-line auction and commerce platform Mercado Libre in Latin America, and the Chinese technology giants Alibaba and Tencent.
These entities now do virtually everything related to finance. Amazon extends loans to small and medium-size businesses. Kakao offers the full range of banking services. Alibaba’s Ant Financial and Tencent’s WeChat provide a cornucopia of financial products, having expanded so rapidly that they recently became targets of a Chinese government crackdown.
The challenges for regulators are obvious. Where a single company channels payments for the majority of a country’s population, as does M-Pesa in Kenya, for example, its failure could crash the entire economy. Regulators must therefore pay close attention to operational risks. They must worry about the protection of customer data – not just financial data but also other personal data to which Big Tech companies are privy.
Moreover, the Big Tech firms, because of their ability to harvest and analyze data on consumer preferences, have an enhanced ability to target their customers’ behavioral biases. If those biases cause some borrowers to take on excessive risk, Big Tech will have little reason to care if it is merely providing technology and expertise to a partner bank. This moral hazard is why Chinese regulators now require the country’s Big Techs to use their own balance sheets to fund 30% of any loan extended via co-lending partnerships.
Governments also have laws and regulations to prevent providers of financial products from discriminating on the basis of race, gender, ethnicity, and religion. The challenge here is distinguishing between price discrimination based on group characteristics and price discrimination based on risk.
Traditionally, regulators require credit providers to list the variables that form the basis for lending decisions so that the regulators can determine whether the variables include prohibited group characteristics. And they require lenders to specify the weights attached to the variables so that they can establish whether lending decisions are uncorrelated with ethnic or racial characteristics once conditioned on those other measures. But as Big Tech companies’ artificial intelligence-based algorithms replace loan officers, the variables and weights will be changing continuously with the arrival of new data points. It’s not obvious that regulators can keep up.
In algorithmic processes, moreover, the source of bias can vary. The data used to train the algorithm may be biased. Alternatively, the training itself may be biased, with the AI algorithm “learning” to use the data in biased ways. Given the black-box nature of algorithmic processes, the location of the problem is rarely clear.
Finally, there are risks to competition. Banks and fintechs rely on cloud computing services operated by the Big Tech firms, rendering them dependent on their most formidable competitors. Big Techs can also cross-subsidize their financial businesses, which are only a small part of what they do. By providing a range of interlocking services, they can prevent their customers from switching providers.
Regulators have responded with open banking rules requiring financial firms to share their customer data with third parties when customers consent. They have authorized the use of application programming interfaces that allow third-party providers to plug directly into financial websites to obtain customer data.
It is not clear that this is enough. Big Techs can use their platforms to generate large amounts of customer data, employ it in training their AI algorithms, and identify high-quality loans more efficiently than competitors lacking the same information. Customers may be able to move their financial data to another bank or fintech, but what about their nonfinancial data? What about the algorithm that has been trained up using one’s data and that of other customers? Without this, digital banks and fintechs won’t be able to price and target their services as efficiently as the Big Techs. Problems of consumer lock-in and market dominance won’t be overcome.
In an old parable about banks and regulators, the banks are greyhounds – they run very fast. The regulators are bloodhounds, slow afoot but faithfully on the trail. In the age of the platform economy, the bloodhounds are going to have to pick up the pace. Given that only three central banks report having dedicated fintech departments, there is reason to worry that they will lose the scent.
Copyright: Project Syndicate, 2021.
a global affairs media network
The Challenge of Big Tech Finance
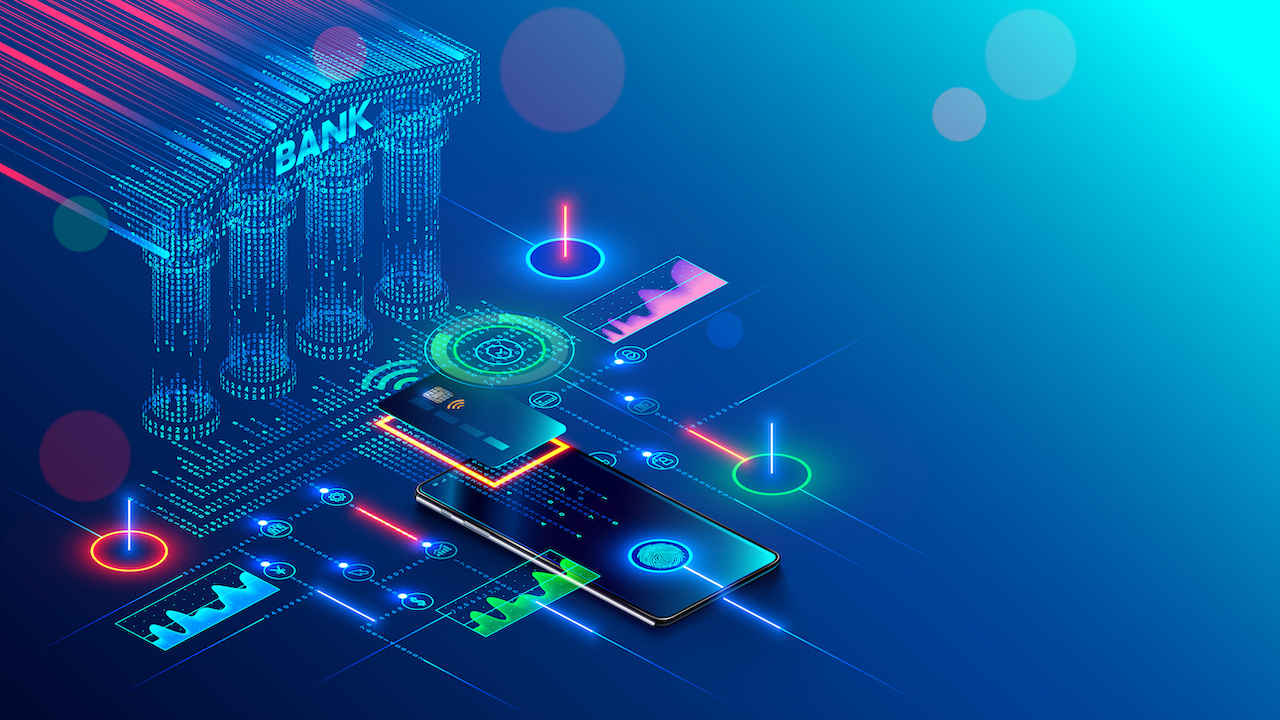
Illustration via AdobeStock.
April 15, 2021
In an old parable about banks and regulators, the banks are greyhounds – they run very fast – while the regulators are bloodhounds, slow afoot but faithfully on the trail. In the age of the platform economy, the bloodhounds are at risk of losing the scent.
I
n 2009, in the midst of the global financial crisis, Paul Volcker, the former Federal Reserve chair, famously observed that the only socially productive financial innovation of the preceding 20 years was the automated teller machine. One wonders what Volcker would make of the tsunami of digitally enabled financial innovations today, from mobile payment platforms to internet banking and peer-to-peer lending.
Volcker might be reassured: like the humble ATM, many of these innovations have tangible benefits in terms of lowering transactions costs. But as a critic of big financial firms, Volcker presumably also would worry about the entry of some very large technology companies into the sector. Their names are as familiar as their services are ubiquitous: e-commerce behemoth Amazon in the United States, messaging company Kakao in Korea, on-line auction and commerce platform Mercado Libre in Latin America, and the Chinese technology giants Alibaba and Tencent.
These entities now do virtually everything related to finance. Amazon extends loans to small and medium-size businesses. Kakao offers the full range of banking services. Alibaba’s Ant Financial and Tencent’s WeChat provide a cornucopia of financial products, having expanded so rapidly that they recently became targets of a Chinese government crackdown.
The challenges for regulators are obvious. Where a single company channels payments for the majority of a country’s population, as does M-Pesa in Kenya, for example, its failure could crash the entire economy. Regulators must therefore pay close attention to operational risks. They must worry about the protection of customer data – not just financial data but also other personal data to which Big Tech companies are privy.
Moreover, the Big Tech firms, because of their ability to harvest and analyze data on consumer preferences, have an enhanced ability to target their customers’ behavioral biases. If those biases cause some borrowers to take on excessive risk, Big Tech will have little reason to care if it is merely providing technology and expertise to a partner bank. This moral hazard is why Chinese regulators now require the country’s Big Techs to use their own balance sheets to fund 30% of any loan extended via co-lending partnerships.
Governments also have laws and regulations to prevent providers of financial products from discriminating on the basis of race, gender, ethnicity, and religion. The challenge here is distinguishing between price discrimination based on group characteristics and price discrimination based on risk.
Traditionally, regulators require credit providers to list the variables that form the basis for lending decisions so that the regulators can determine whether the variables include prohibited group characteristics. And they require lenders to specify the weights attached to the variables so that they can establish whether lending decisions are uncorrelated with ethnic or racial characteristics once conditioned on those other measures. But as Big Tech companies’ artificial intelligence-based algorithms replace loan officers, the variables and weights will be changing continuously with the arrival of new data points. It’s not obvious that regulators can keep up.
In algorithmic processes, moreover, the source of bias can vary. The data used to train the algorithm may be biased. Alternatively, the training itself may be biased, with the AI algorithm “learning” to use the data in biased ways. Given the black-box nature of algorithmic processes, the location of the problem is rarely clear.
Finally, there are risks to competition. Banks and fintechs rely on cloud computing services operated by the Big Tech firms, rendering them dependent on their most formidable competitors. Big Techs can also cross-subsidize their financial businesses, which are only a small part of what they do. By providing a range of interlocking services, they can prevent their customers from switching providers.
Regulators have responded with open banking rules requiring financial firms to share their customer data with third parties when customers consent. They have authorized the use of application programming interfaces that allow third-party providers to plug directly into financial websites to obtain customer data.
It is not clear that this is enough. Big Techs can use their platforms to generate large amounts of customer data, employ it in training their AI algorithms, and identify high-quality loans more efficiently than competitors lacking the same information. Customers may be able to move their financial data to another bank or fintech, but what about their nonfinancial data? What about the algorithm that has been trained up using one’s data and that of other customers? Without this, digital banks and fintechs won’t be able to price and target their services as efficiently as the Big Techs. Problems of consumer lock-in and market dominance won’t be overcome.
In an old parable about banks and regulators, the banks are greyhounds – they run very fast. The regulators are bloodhounds, slow afoot but faithfully on the trail. In the age of the platform economy, the bloodhounds are going to have to pick up the pace. Given that only three central banks report having dedicated fintech departments, there is reason to worry that they will lose the scent.
Copyright: Project Syndicate, 2021.