.
At 8th annual Privacy Papers for Policy Makers conference in February, the Future of Privacy Forum unveiled the winning Privacy Papers for Policy Makers and recognized leading analytical work in privacy policy. FPF invited scholars and analysts to submit papers for the Privacy Paper for Policymakers Award, which honors leading work relevant to policymakers in the U.S. Congress, at U.S. federal agencies, and for data protection authorities abroad.
The selected papers discuss three major themes on big data: the current lack of sufficient regulation to control the big data boom, the lack of market mechanisms to bring about new security protocols, and the existence of underexplored big data consequences.
Insufficient Big Data Regulation
Several papers chosen point out that current big data legislation is unequal across both populations and nations. Craig Konnoth’s “Health Information Equity”, for example, explores the inequality inherent in using big data for informational medical research. Konnoth argues that although stores of data collected from Medicare records have facilitated a new wave of breakthroughs in research, the data collected places an undue burden on the elderly and sick. Konnoth further argues that there is the risk of identity theft, medical and financial fraud, tainted medical records, and insurance discrimination in every instance of data transmission. Because the sick and elderly are more likely to have data in the collective pot, they bear the brunt of the inherent risks, whereas younger, wealthier and more healthy patients who are less likely to have data in the pot reap the rewards of medical research.
Not only does current legislation allow for unequal distribution of risk, but it facilitates discrimination toward vulnerable populations. In “Algorithmic Jim Crow” Margaret Hu argues that immigrant-related vetting and screening protocols based on new big data capabilities have facilitated the creation of a second wave of Jim Crow Laws. This form of vetting involves using data surveillance to calculate criminal and terroristic risk across entire populations. Hu argues that traditional Jim Crow used a two-step, classification then screening procedure to exclude the African-American population from privileges enjoyed by white citizens. In the age of big data, traditional classification will be replaced by risk assessment, which may then be used to exclude individuals from activities such as air travel. In this way, the heart of risk assessment laws remains in separation and segregation. Therefore, although current legislation purports to be “race neutral”, in reality it allows for systemic discrimination to take place.
Just as current legislation is unequal across populations, it is unequal across nations. In “Transatlantic Data Privacy Law” by Paul Schwartz and Karl-Nikolaus Peifer, the authors outline the differences between data privacy laws in the EU and in the United States, as well as the problems these differences create for multinational corporations. The EU privacy laws, which are dictated by the European Directive on Data Collection and the General Data Protection Regulation, are generally stricter than U.S. privacy laws. The EU prevents third party nations without strict enough privacy laws to use its data, a mandate that revolves around a privacy culture based on individuals’ basic human right to privacy. The United States, on the other hand, treats individuals as private consumers and critiques EU law as protectionist. As a result, the United States has very little Constitutional protection. This disparity has given rise to the so-called Transatlantic Data War, or the dispute between the EU and the United States over EU perception that U.S. companies utilizing EU private data for services lack sufficient privacy laws.
In light of this disparity Samson Esayas advocates for the EU to adopt a more holistic approach to privacy protection in “The Idea of ‘Emergent Properties’ in Data Privacy: A Holistic Approach”. Esayas argues that the EU individualistic approach to data processing is unrealistic in today’s big data climate. Instead, the EU should implement a holistic approach in which market actors are given greater privacy responsibility on a case-by-case basis after reaching a threshold number of channels.
Big data regulation has also failed to sufficiently operationalize its core concepts. In his article, “The Public Information Fallacy” Woodrow Hartzog points out that although privacy laws are built on the concept of public v. private information, there is no universally accepted definition of what the term “public” means. Hartzog argues in the legislation alone, there are three ways to conceptualize public: as descriptive context or content, as anything that isn’t private, and as information specifically deemed public by an authoritative force like the government. Because there isn’t one way to conceptualize what “public” is, data consumers’ privacy protection may be undermined. For example, the FBI alleged it doesn’t need permission to conduct surveillance using powerful technologies like cell-site simulators (often called Stingrays), so long as they are in public places; however, because “public” is so vaguely defined, law enforcement has the opportunity to misuse data collection practices.
Finally, Ryan Calo argues in “Artificial Intelligence Policy: A Primer and Roadmap” that the artificial intelligence landscape that has emerged in the last decade carries with it the need for new, extensive regulation. He outlines a series of necessary questions for policy maker pertaining to inequality in the application of AI, whether AI should be used for consequential decision making, the use of force, safety thresholds, certification, and privacy.
Lack of Market Mechanisms
As each of the papers chosen to participate in the 2018 FFP have made clear, big data processing comes with inherent risks; however, there is very little universal security protocol in place to protect consumers. Along these lines in “Private Infrastructure and the Internet of Things: The Case of Automobiles” Deirdre Mulligan and Kenneth Bamberger use the auto industry as a microcosm to demonstrate how the adoption of big data capabilities by manufacturers has not been accompanied by necessary security. Mulligan and Bamberger point out that that code in cars, especially in their safety systems, and in the broader range of objects—such as cellular phones—that interact with them present new opportunities for failure and malfeasance. Despite these risks, there is no universal or sufficient standard for code security. Mulligan and Bamberger explain this lack of security by arguing that cyber security is effectively a public good that requires government action. Therefore, only forced over the air (OTA) updates can address the systemic lack of security that currently exists in “smart technology”.
Similar to Mulligan and Bamberger, Chetan Gupta explores the lack of market mechanisms to incentivize corporations and manufacturers to improve cyber security in “The Market’s Law of Privacy: Case Studies in Privacy/Security Adoption”. Gupta uses a series of case studies on security adoption to demonstrate that single actors/groups that do drive the adoption of a standard are significant players in the industry. As major players adopt new security protocol it becomes market standard, leading other market participants to adopt it regardless of cost. For example, Hypertext Transfer Protocol Secure (HTTPS), a protocol that encrypts data and is designed to withstand attacks, was relatively unused until Google announced its plans to adopt HTTPS in August of 2014. Immediately afterward there was a rapid uptake in HTTPS by other market participants. Gupta predicts that a similar process will take place with respect to Extended Validation (EV) SSL certificates and Certificate Transparency (CT), which is a verification process that necessitates an entity “to prove exclusive rights to use a domain, confirm it’s legal, operational and with physical existence, and prove the entity has authorized the issuance of the Certificate.” Google published a new initiative adopting these protocols in 2016 and Facebook has strongly advocated for them.
Underexplored Consequences of Using Big Data
Both private industry and government have wholly embraced big Data processes; however, the consequences of utilizing big data remain relatively unexplored. These consequences provided an ominous undercurrent to the 2018 PPPM papers. In “The Undue Influence of Surveillance Technology Companies on Policing” Elizabeth Joh explores how big data technologies marketed toward police enforcement in the United States has conveyed certain companies an overly large influence over law enforcement agencies. Police enforcement have recently adopted stingrays, or cell-site simulators sold exclusively by Harris Corporation, body cameras sold by Taser, and big data software. Because of this, Harris Corporation and Taser, through nondisclosure agreements and proprietary information, exert influence over law enforcement’s actions. Specifically, they prevent law enforcement from disclosing relevant information normally disclosed to defendants and the public. This influence has long-reaching negative consequences, including distortion of the Fourth Amendment, placement of undue suspicion on groups, oversight of disclosing information to local communities and public record, and lack of transparency.
Discrimination on online forums, too, may be a consequence of big data processes. In “Designing Against Discrimination in Online Markets” Karen Levy and Solon Barocas argue that the availability of descriptive personal information online facilitates online discrimination. Although corporations increasingly have implemented sensitivity training, in light of the explosion of big data processes they must also be aware of how they structure the community compositions, policies, interaction structuring, and monitoring and evaluation protocol to better address discrimination. “Algorithmic Jim Crow” also demonstrates how big data process may facilitate inadvertently systemic discrimination.
The views presented in this article are the author’s own and do not necessarily represent the views of any other organization.
a global affairs media network
The Big Debate on Privacy in Big Data
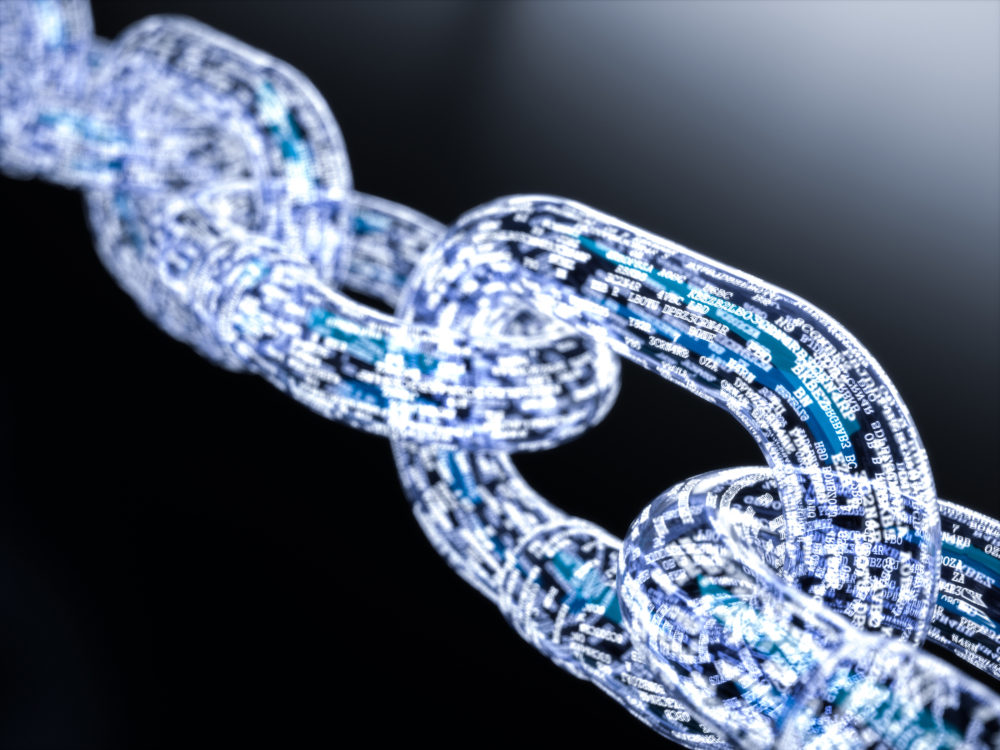
Blockchain digital illuminated shape. Big data node
April 30, 2018
At 8th annual Privacy Papers for Policy Makers conference in February, the Future of Privacy Forum unveiled the winning Privacy Papers for Policy Makers and recognized leading analytical work in privacy policy. FPF invited scholars and analysts to submit papers for the Privacy Paper for Policymakers Award, which honors leading work relevant to policymakers in the U.S. Congress, at U.S. federal agencies, and for data protection authorities abroad.
The selected papers discuss three major themes on big data: the current lack of sufficient regulation to control the big data boom, the lack of market mechanisms to bring about new security protocols, and the existence of underexplored big data consequences.
Insufficient Big Data Regulation
Several papers chosen point out that current big data legislation is unequal across both populations and nations. Craig Konnoth’s “Health Information Equity”, for example, explores the inequality inherent in using big data for informational medical research. Konnoth argues that although stores of data collected from Medicare records have facilitated a new wave of breakthroughs in research, the data collected places an undue burden on the elderly and sick. Konnoth further argues that there is the risk of identity theft, medical and financial fraud, tainted medical records, and insurance discrimination in every instance of data transmission. Because the sick and elderly are more likely to have data in the collective pot, they bear the brunt of the inherent risks, whereas younger, wealthier and more healthy patients who are less likely to have data in the pot reap the rewards of medical research.
Not only does current legislation allow for unequal distribution of risk, but it facilitates discrimination toward vulnerable populations. In “Algorithmic Jim Crow” Margaret Hu argues that immigrant-related vetting and screening protocols based on new big data capabilities have facilitated the creation of a second wave of Jim Crow Laws. This form of vetting involves using data surveillance to calculate criminal and terroristic risk across entire populations. Hu argues that traditional Jim Crow used a two-step, classification then screening procedure to exclude the African-American population from privileges enjoyed by white citizens. In the age of big data, traditional classification will be replaced by risk assessment, which may then be used to exclude individuals from activities such as air travel. In this way, the heart of risk assessment laws remains in separation and segregation. Therefore, although current legislation purports to be “race neutral”, in reality it allows for systemic discrimination to take place.
Just as current legislation is unequal across populations, it is unequal across nations. In “Transatlantic Data Privacy Law” by Paul Schwartz and Karl-Nikolaus Peifer, the authors outline the differences between data privacy laws in the EU and in the United States, as well as the problems these differences create for multinational corporations. The EU privacy laws, which are dictated by the European Directive on Data Collection and the General Data Protection Regulation, are generally stricter than U.S. privacy laws. The EU prevents third party nations without strict enough privacy laws to use its data, a mandate that revolves around a privacy culture based on individuals’ basic human right to privacy. The United States, on the other hand, treats individuals as private consumers and critiques EU law as protectionist. As a result, the United States has very little Constitutional protection. This disparity has given rise to the so-called Transatlantic Data War, or the dispute between the EU and the United States over EU perception that U.S. companies utilizing EU private data for services lack sufficient privacy laws.
In light of this disparity Samson Esayas advocates for the EU to adopt a more holistic approach to privacy protection in “The Idea of ‘Emergent Properties’ in Data Privacy: A Holistic Approach”. Esayas argues that the EU individualistic approach to data processing is unrealistic in today’s big data climate. Instead, the EU should implement a holistic approach in which market actors are given greater privacy responsibility on a case-by-case basis after reaching a threshold number of channels.
Big data regulation has also failed to sufficiently operationalize its core concepts. In his article, “The Public Information Fallacy” Woodrow Hartzog points out that although privacy laws are built on the concept of public v. private information, there is no universally accepted definition of what the term “public” means. Hartzog argues in the legislation alone, there are three ways to conceptualize public: as descriptive context or content, as anything that isn’t private, and as information specifically deemed public by an authoritative force like the government. Because there isn’t one way to conceptualize what “public” is, data consumers’ privacy protection may be undermined. For example, the FBI alleged it doesn’t need permission to conduct surveillance using powerful technologies like cell-site simulators (often called Stingrays), so long as they are in public places; however, because “public” is so vaguely defined, law enforcement has the opportunity to misuse data collection practices.
Finally, Ryan Calo argues in “Artificial Intelligence Policy: A Primer and Roadmap” that the artificial intelligence landscape that has emerged in the last decade carries with it the need for new, extensive regulation. He outlines a series of necessary questions for policy maker pertaining to inequality in the application of AI, whether AI should be used for consequential decision making, the use of force, safety thresholds, certification, and privacy.
Lack of Market Mechanisms
As each of the papers chosen to participate in the 2018 FFP have made clear, big data processing comes with inherent risks; however, there is very little universal security protocol in place to protect consumers. Along these lines in “Private Infrastructure and the Internet of Things: The Case of Automobiles” Deirdre Mulligan and Kenneth Bamberger use the auto industry as a microcosm to demonstrate how the adoption of big data capabilities by manufacturers has not been accompanied by necessary security. Mulligan and Bamberger point out that that code in cars, especially in their safety systems, and in the broader range of objects—such as cellular phones—that interact with them present new opportunities for failure and malfeasance. Despite these risks, there is no universal or sufficient standard for code security. Mulligan and Bamberger explain this lack of security by arguing that cyber security is effectively a public good that requires government action. Therefore, only forced over the air (OTA) updates can address the systemic lack of security that currently exists in “smart technology”.
Similar to Mulligan and Bamberger, Chetan Gupta explores the lack of market mechanisms to incentivize corporations and manufacturers to improve cyber security in “The Market’s Law of Privacy: Case Studies in Privacy/Security Adoption”. Gupta uses a series of case studies on security adoption to demonstrate that single actors/groups that do drive the adoption of a standard are significant players in the industry. As major players adopt new security protocol it becomes market standard, leading other market participants to adopt it regardless of cost. For example, Hypertext Transfer Protocol Secure (HTTPS), a protocol that encrypts data and is designed to withstand attacks, was relatively unused until Google announced its plans to adopt HTTPS in August of 2014. Immediately afterward there was a rapid uptake in HTTPS by other market participants. Gupta predicts that a similar process will take place with respect to Extended Validation (EV) SSL certificates and Certificate Transparency (CT), which is a verification process that necessitates an entity “to prove exclusive rights to use a domain, confirm it’s legal, operational and with physical existence, and prove the entity has authorized the issuance of the Certificate.” Google published a new initiative adopting these protocols in 2016 and Facebook has strongly advocated for them.
Underexplored Consequences of Using Big Data
Both private industry and government have wholly embraced big Data processes; however, the consequences of utilizing big data remain relatively unexplored. These consequences provided an ominous undercurrent to the 2018 PPPM papers. In “The Undue Influence of Surveillance Technology Companies on Policing” Elizabeth Joh explores how big data technologies marketed toward police enforcement in the United States has conveyed certain companies an overly large influence over law enforcement agencies. Police enforcement have recently adopted stingrays, or cell-site simulators sold exclusively by Harris Corporation, body cameras sold by Taser, and big data software. Because of this, Harris Corporation and Taser, through nondisclosure agreements and proprietary information, exert influence over law enforcement’s actions. Specifically, they prevent law enforcement from disclosing relevant information normally disclosed to defendants and the public. This influence has long-reaching negative consequences, including distortion of the Fourth Amendment, placement of undue suspicion on groups, oversight of disclosing information to local communities and public record, and lack of transparency.
Discrimination on online forums, too, may be a consequence of big data processes. In “Designing Against Discrimination in Online Markets” Karen Levy and Solon Barocas argue that the availability of descriptive personal information online facilitates online discrimination. Although corporations increasingly have implemented sensitivity training, in light of the explosion of big data processes they must also be aware of how they structure the community compositions, policies, interaction structuring, and monitoring and evaluation protocol to better address discrimination. “Algorithmic Jim Crow” also demonstrates how big data process may facilitate inadvertently systemic discrimination.
The views presented in this article are the author’s own and do not necessarily represent the views of any other organization.