here has been rapid adoption of generative AI (GenAI) in the past 11 months, and this trend is expected to grow in the coming years. Many companies are betting their worth on the Large Language Models (LLMs) like Google with Bard (LaMDA); Meta with Llama; Microsoft and Open AI with GPT; Amazon and Anthropic with Claude. As competition gets fierce, the economics of the LLM applications will become a deciding factor for the success of AI enterprises.
Developing and deploying the software infrastructure needed to support GenAI deployments—including frameworks, libraries, and APIs—is a meticulous task that requires continuous updates and management. GenAI models, especially those involving deep learning, require significant computational power. Training and hyperparameter tuning such models often demand high-performance graphical processing units (GPUs) which can be expensive. Deployments, on the other hand, rely on distributed computing to handle computational demands. Effectively managing these distributed systems adds another layer of complexity regarding coordination, data synchronization, and network communication.
Large enterprises with deeper investments will focus on the commercialization of Generative AI applications while staying within the regulatory guardrails—a challenging but imperative task. Enterprises across industries operating at the largest scales consisting of terabytes of data will start focusing on productizing generative AI applications through centralized Generative AI CoE. The Generative AI Center of Excellence (CoE) can aid this process of enabling productivity and monetization. With the rapid adoption of Generative AI across various industries, computing will become scarce. Securing GPUs at scale will need significant investment from the enterprise. These investments can be reduced to a fraction if these LLMs are centrally trained, hyperparameter tuned, and made available for downstream teams through robust built-in pipelines and platforms. Technology enterprises will soon shift their focus from research to productization and monetization of Generative AI. There will also be a significant emphasis on algorithmic safety as new regulations around responsible AI, explainability, and interpretability are rolled out—adding guardrails around monetizing these solutions in a socially responsible manner, making the ecosystem more complex.
Monetizing Generative AI technology within safety guardrails will be a massive focus for all players in the coming years. Through centralized productization of Gen AI capabilities, enterprises will grow optimistic in enhancing revenue, fostering innovation, and enabling technology accessibility globally.
a global affairs media network
Commercializing and Monetizing Generative AI
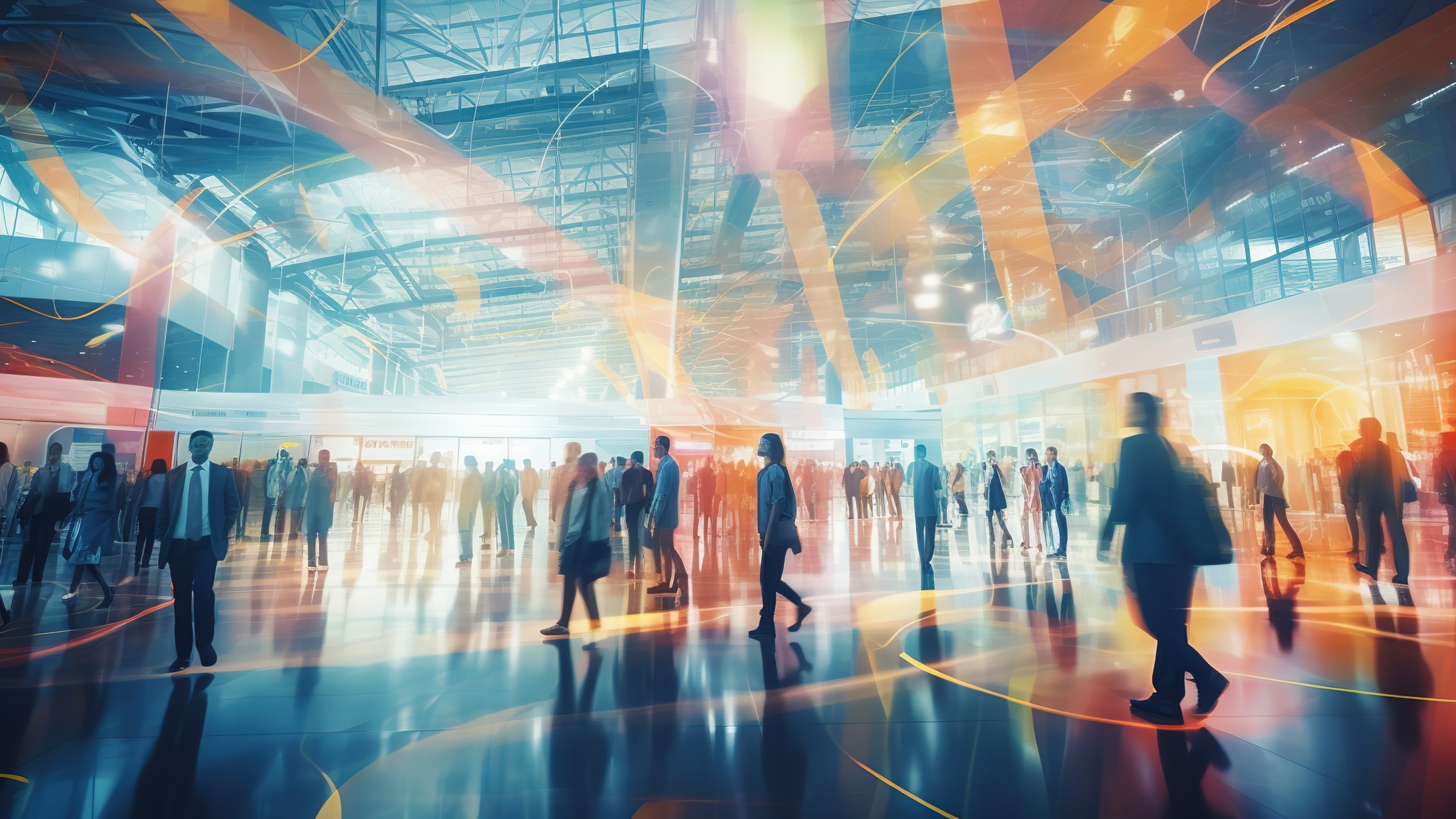
Image via Adobe Stock.
January 17, 2024
Adoption of generative AI will continue to accelerate in the coming years, and competition among developers will likely intensify. Monetizing generative AI within regulatory guardrails will be a challenging but imperative focus of developers in the coming years, writes Srujana Kaddervarmuth.
T
here has been rapid adoption of generative AI (GenAI) in the past 11 months, and this trend is expected to grow in the coming years. Many companies are betting their worth on the Large Language Models (LLMs) like Google with Bard (LaMDA); Meta with Llama; Microsoft and Open AI with GPT; Amazon and Anthropic with Claude. As competition gets fierce, the economics of the LLM applications will become a deciding factor for the success of AI enterprises.
Developing and deploying the software infrastructure needed to support GenAI deployments—including frameworks, libraries, and APIs—is a meticulous task that requires continuous updates and management. GenAI models, especially those involving deep learning, require significant computational power. Training and hyperparameter tuning such models often demand high-performance graphical processing units (GPUs) which can be expensive. Deployments, on the other hand, rely on distributed computing to handle computational demands. Effectively managing these distributed systems adds another layer of complexity regarding coordination, data synchronization, and network communication.
Large enterprises with deeper investments will focus on the commercialization of Generative AI applications while staying within the regulatory guardrails—a challenging but imperative task. Enterprises across industries operating at the largest scales consisting of terabytes of data will start focusing on productizing generative AI applications through centralized Generative AI CoE. The Generative AI Center of Excellence (CoE) can aid this process of enabling productivity and monetization. With the rapid adoption of Generative AI across various industries, computing will become scarce. Securing GPUs at scale will need significant investment from the enterprise. These investments can be reduced to a fraction if these LLMs are centrally trained, hyperparameter tuned, and made available for downstream teams through robust built-in pipelines and platforms. Technology enterprises will soon shift their focus from research to productization and monetization of Generative AI. There will also be a significant emphasis on algorithmic safety as new regulations around responsible AI, explainability, and interpretability are rolled out—adding guardrails around monetizing these solutions in a socially responsible manner, making the ecosystem more complex.
Monetizing Generative AI technology within safety guardrails will be a massive focus for all players in the coming years. Through centralized productization of Gen AI capabilities, enterprises will grow optimistic in enhancing revenue, fostering innovation, and enabling technology accessibility globally.