.
Artificial Intelligence (AI) may well be the most powerful technology of the 21st century, helping to solve humanity’s most complex unsolved problems: environmental, social, and more. Yet sceptics believe that AI’s risks are as large as its potential benefits. How can they be avoided? And why isn’t the most powerful technology being used more widely today to solve the world’s greatest “wicked” problems?
Great technological advances are often a double-edged sword. A backlash follows an initial “honeymoon period”. Unintended consequences that were not immediately obvious during the technology’s infancy become more apparent, and may even overwhelm the initial, benefits. A historical example is the cotton gin, which led indirectly to the U.S. Civil War. The challenge is to iron out the rough edges while preserving the benefits.
We have been living through a great technological revolution, driven by the ever-increasing capabilities of information systems, and convergence with communications technology. The byproduct is the widespread availability of unimaginably vast amounts of data.
Yet data value is latent at best, unless we have some way to make sense of it. This is where AI fits in: it processes data to produce insights. Yet, today, the “AI honeymoon” is coming to an end, experiencing the first waves of widely recognized unintended consequences.
Gathering and analyzing data is, in its way, a new kind of “microscope”, providing insights into human behavior, science, medicine, and more. For instance, a Target grocery store can now famously determine if a girl is pregnant before her parents find out. And the recent controversy around the exploitation of Facebook’s microtargeting capabilities to propagate fake news, with impacts on elections worldwide, raises the prospect that democratic outcomes will be undermined by AI-driven voter-manipulation campaigns. Some even predict that AI poses an existential threat to humanity.
So how can we avoid the negatives of AI while maximizing its benefits? Here are some guidelines:
Understand that AI today is limited. By far, the majority of successful AI scenarios involve a single “link”. “Here’s a picture of a city street, what does it show?”, “Here’s Joe’s Amazon browsing and purchasing history, what new product will Joe he want next?”, “Here’s a voter, what issues are they most interested in?”, “Here’s information about a medical device, when will it fail”?
Know that AI is subject to dangerous biases. What if, for example, an AI system were to identify that persons of a certain ethnicity were more prone to violence, and therefore should not be allowed into certain housing projects? This may have nothing to do with the real traits of any particular ethnic group, but rather with unseen idiosyncrasies in the training data. But, the AI system can’t tell the difference.
The pattern here is insidious: the correlation between race and violence may be well-established in a given data set, but that doesn’t mean that race is the causative factor for the violence: the cause could be historical events that happen to correlate with race.
AI systems do not “understand” the world. They don’t find causal connections, only correlations in the data they are presented with. The solution to endemic violence isn’t found by excluding persons of a certain race, but rather by removing the underlying factors that have disproportionately affected people of a certain race.
To do this, the near-term future of AI is to map causal links to form a chain from actions to outcomes, and to show how two correlating things may not cause each other “If I invest $X in police and $Y in the legal system, how will that impact crime?”, “If I visit voters in this neighborhood, how much will that help my candidate to be elected?” And multi-link thinking is essential to solving the next set of hard problems in a complex world.
More often than not, these links involve “soft” factors, like attitude, empathy, morale, hope, and fear.
These chains of events often contain loops that build on each other, in “vicious” and “virtuous” cycles, as shown below. For instance: “Money invested in policing and legal services lead to increased trust in the government, which leads to decreased preemptive violence, which leads to social stability, which leads to business investment, which increases the tax base, which gives us more money for policing and legal services.”, “As more and more of our workforce in this area is sick, they are less able to work, decreasing the tax base, and reducing our ability to invest in health care.”
AI alone is not able to determine how these cause-and-effect chains fit together. That requires human expertise, in a hybrid “augmented intelligence” scenario.
Data today is pervasive, and AI helps brings value to it. “Multi-link” AI―called Decision Intelligence (DI)―solves the hard AI problems. To reap the greatest benefit from AI, while avoiding its negatives, we need to evolve beyond inward-facing, single-link systems, and use technology to model, as well, the complex causal systems in which they function.
About the author: A 35-year AI veteran, Pratt is Chief Scientist at Quantellia, which builds AI/DI systems worldwide, and is cofounder at winworks.ai, which bridges candidates to their constituents. Pratt was recently recognized as an outstanding Woman Innovator and is known for her pioneering work on transfer learning and decision intelligence.
Editor's Note: This article was originally published in the print edition of the 2018 G7 Summit magazine.

The views presented in this article are the author’s own and do not necessarily represent the views of any other organization.
a global affairs media network
Artificial Intelligence: The Miracle and the Menace
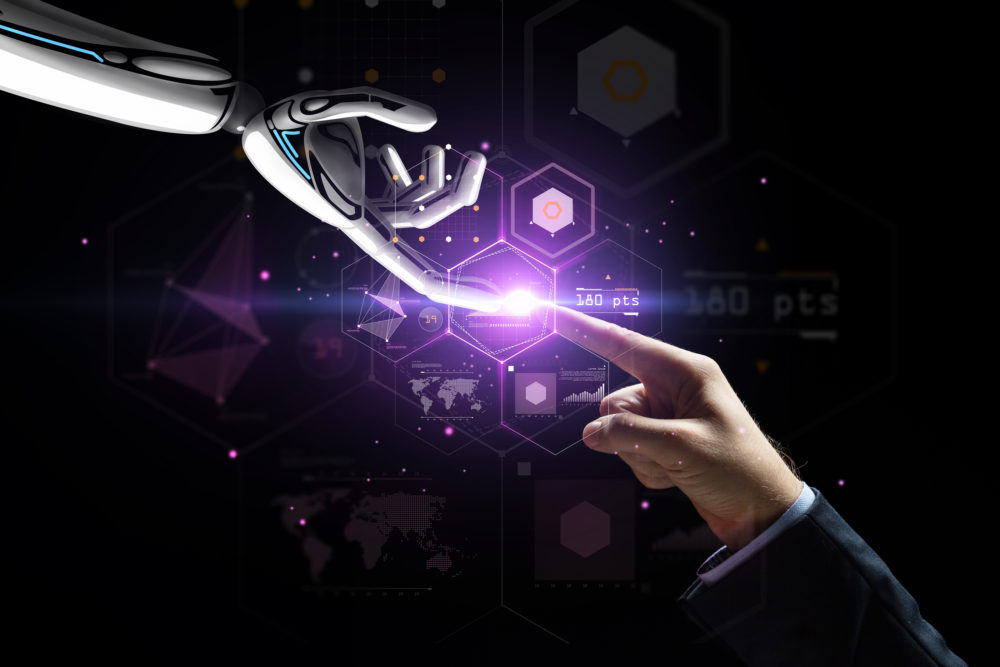
artificial intelligence, future technology and business concept |
June 1, 2018
Artificial Intelligence (AI) may well be the most powerful technology of the 21st century, helping to solve humanity’s most complex unsolved problems: environmental, social, and more. Yet sceptics believe that AI’s risks are as large as its potential benefits. How can they be avoided? And why isn’t the most powerful technology being used more widely today to solve the world’s greatest “wicked” problems?
Great technological advances are often a double-edged sword. A backlash follows an initial “honeymoon period”. Unintended consequences that were not immediately obvious during the technology’s infancy become more apparent, and may even overwhelm the initial, benefits. A historical example is the cotton gin, which led indirectly to the U.S. Civil War. The challenge is to iron out the rough edges while preserving the benefits.
We have been living through a great technological revolution, driven by the ever-increasing capabilities of information systems, and convergence with communications technology. The byproduct is the widespread availability of unimaginably vast amounts of data.
Yet data value is latent at best, unless we have some way to make sense of it. This is where AI fits in: it processes data to produce insights. Yet, today, the “AI honeymoon” is coming to an end, experiencing the first waves of widely recognized unintended consequences.
Gathering and analyzing data is, in its way, a new kind of “microscope”, providing insights into human behavior, science, medicine, and more. For instance, a Target grocery store can now famously determine if a girl is pregnant before her parents find out. And the recent controversy around the exploitation of Facebook’s microtargeting capabilities to propagate fake news, with impacts on elections worldwide, raises the prospect that democratic outcomes will be undermined by AI-driven voter-manipulation campaigns. Some even predict that AI poses an existential threat to humanity.
So how can we avoid the negatives of AI while maximizing its benefits? Here are some guidelines:
Understand that AI today is limited. By far, the majority of successful AI scenarios involve a single “link”. “Here’s a picture of a city street, what does it show?”, “Here’s Joe’s Amazon browsing and purchasing history, what new product will Joe he want next?”, “Here’s a voter, what issues are they most interested in?”, “Here’s information about a medical device, when will it fail”?
Know that AI is subject to dangerous biases. What if, for example, an AI system were to identify that persons of a certain ethnicity were more prone to violence, and therefore should not be allowed into certain housing projects? This may have nothing to do with the real traits of any particular ethnic group, but rather with unseen idiosyncrasies in the training data. But, the AI system can’t tell the difference.
The pattern here is insidious: the correlation between race and violence may be well-established in a given data set, but that doesn’t mean that race is the causative factor for the violence: the cause could be historical events that happen to correlate with race.
AI systems do not “understand” the world. They don’t find causal connections, only correlations in the data they are presented with. The solution to endemic violence isn’t found by excluding persons of a certain race, but rather by removing the underlying factors that have disproportionately affected people of a certain race.
To do this, the near-term future of AI is to map causal links to form a chain from actions to outcomes, and to show how two correlating things may not cause each other “If I invest $X in police and $Y in the legal system, how will that impact crime?”, “If I visit voters in this neighborhood, how much will that help my candidate to be elected?” And multi-link thinking is essential to solving the next set of hard problems in a complex world.
More often than not, these links involve “soft” factors, like attitude, empathy, morale, hope, and fear.
These chains of events often contain loops that build on each other, in “vicious” and “virtuous” cycles, as shown below. For instance: “Money invested in policing and legal services lead to increased trust in the government, which leads to decreased preemptive violence, which leads to social stability, which leads to business investment, which increases the tax base, which gives us more money for policing and legal services.”, “As more and more of our workforce in this area is sick, they are less able to work, decreasing the tax base, and reducing our ability to invest in health care.”
AI alone is not able to determine how these cause-and-effect chains fit together. That requires human expertise, in a hybrid “augmented intelligence” scenario.
Data today is pervasive, and AI helps brings value to it. “Multi-link” AI―called Decision Intelligence (DI)―solves the hard AI problems. To reap the greatest benefit from AI, while avoiding its negatives, we need to evolve beyond inward-facing, single-link systems, and use technology to model, as well, the complex causal systems in which they function.
About the author: A 35-year AI veteran, Pratt is Chief Scientist at Quantellia, which builds AI/DI systems worldwide, and is cofounder at winworks.ai, which bridges candidates to their constituents. Pratt was recently recognized as an outstanding Woman Innovator and is known for her pioneering work on transfer learning and decision intelligence.
Editor's Note: This article was originally published in the print edition of the 2018 G7 Summit magazine.

The views presented in this article are the author’s own and do not necessarily represent the views of any other organization.