he global race for artificial intelligence dominance isn't just about having more AI professionals, it's about having the right ones. Behind the headlines about a growing AI workforce lies a critical insight missing from policy discussions: all AI talent is not the same. The technical composition of AI talent varies dramatically across ecosystems, and understanding these differences is essential for nurturing and attracting the right talent.
Research suggests that long–standing AI ecosystems may naturally diversify toward business and implementation roles. Only 10% of the U.S. AI workforce possesses the advanced deep learning expertise necessary to build sophisticated AI systems. In contrast, countries like South Korea (27%), Israel (23%), and Japan (20%) maintain significantly higher proportions of specialized technical talent in their AI workforces. Among European contenders, Poland (20%) and Germany (18%) rank 4th and 5th globally, potentially emerging as future AI development powerhouses.
Perhaps most revealing is that nearly all countries have equal or higher percentages of foreign–trained deep learning experts than domestic ones. This highlights a crucial reality: immigration policies may be as important as educational investments for building technological sovereignty.
The past year has witnessed an unprecedented exodus of specialized AI researchers from American institutions, creating a "brain drain" precisely when such expertise has become crucial for national competitiveness. Given the profound mismatch between market demand and current workforce composition, the UK is preparing to launch a £50 million scheme "to woo international research talent," focusing on priority areas including artificial intelligence. Similar initiatives are emerging across Europe, with France launching a "Safe Place for Science" campaign allocating €15 million to fund American scientists considering "scientific exile." These coordinated international efforts highlight the growing recognition that AI expertise has become a strategic national resource.
The most effective AI talent development approaches are targeted. Generic "AI upskilling" programs often miss the mark because different skill levels require tailored strategies. Consider this simple example: training someone with basic AI literacy to become a technical expert requires different content than developing a technical expert into a deep specialist. Teaching advanced neural network architecture to someone who barely understands algorithms would be ineffective, while basic introductory courses would waste time for those ready to innovate.
To address this complexity, I've developed a three–tier classification system: AI literacy (basic understanding), technical expertise (implementation capabilities), and deep specialization (innovation capacity). This framework helps organizations develop targeted interventions that match their specific needs.
For policymakers, the implications are clear: successful AI strategies must simultaneously develop domestic educational pipelines while creating environments that attract and retain specialized global talent. For business leaders, this means developing internal pathways that strategically upskill talent based on specific organizational needs rather than pursuing generic development.
As specialized talent flows reshape along geopolitical lines, nations that implement data–informed, targeted talent development strategies will gain a competitive edge. Success depends on both nurturing domestic expertise and creating environments that attract global innovators, moving beyond generic approaches to address the specific needs of each AI ecosystem.
a global affairs media network
Advanced AI expert migration: Small talent pool, global impact
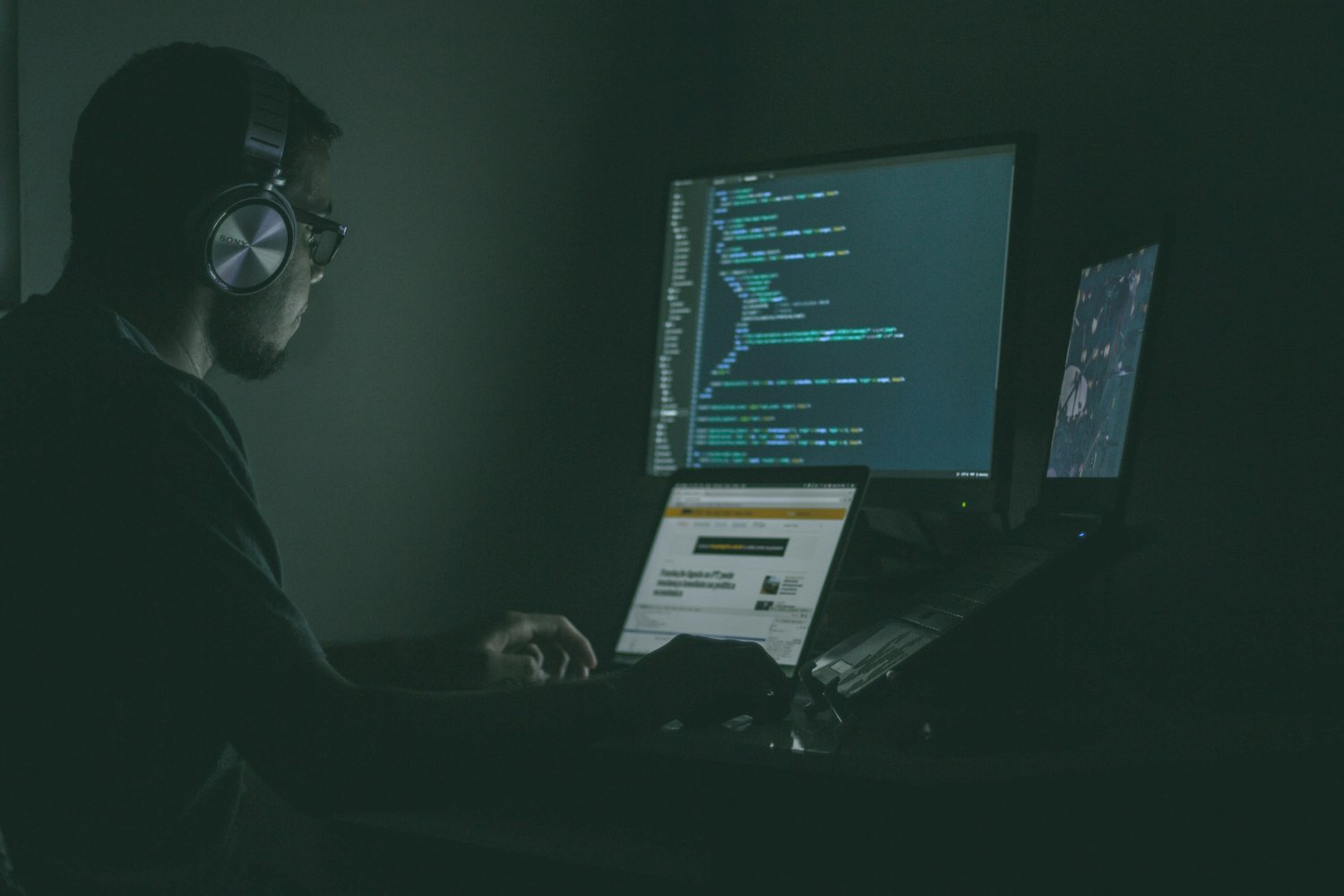
hoto by Jefferson Santos on Unsplash
May 28, 2025
As countries compete for AI dominance, doing well requires not just having more AI professionals but increasingly, having the right ones. As specialized talent flows reshape along geopolitical lines, successful nations will target talent development strategies that move beyond generic approaches, wr
T
he global race for artificial intelligence dominance isn't just about having more AI professionals, it's about having the right ones. Behind the headlines about a growing AI workforce lies a critical insight missing from policy discussions: all AI talent is not the same. The technical composition of AI talent varies dramatically across ecosystems, and understanding these differences is essential for nurturing and attracting the right talent.
Research suggests that long–standing AI ecosystems may naturally diversify toward business and implementation roles. Only 10% of the U.S. AI workforce possesses the advanced deep learning expertise necessary to build sophisticated AI systems. In contrast, countries like South Korea (27%), Israel (23%), and Japan (20%) maintain significantly higher proportions of specialized technical talent in their AI workforces. Among European contenders, Poland (20%) and Germany (18%) rank 4th and 5th globally, potentially emerging as future AI development powerhouses.
Perhaps most revealing is that nearly all countries have equal or higher percentages of foreign–trained deep learning experts than domestic ones. This highlights a crucial reality: immigration policies may be as important as educational investments for building technological sovereignty.
The past year has witnessed an unprecedented exodus of specialized AI researchers from American institutions, creating a "brain drain" precisely when such expertise has become crucial for national competitiveness. Given the profound mismatch between market demand and current workforce composition, the UK is preparing to launch a £50 million scheme "to woo international research talent," focusing on priority areas including artificial intelligence. Similar initiatives are emerging across Europe, with France launching a "Safe Place for Science" campaign allocating €15 million to fund American scientists considering "scientific exile." These coordinated international efforts highlight the growing recognition that AI expertise has become a strategic national resource.
The most effective AI talent development approaches are targeted. Generic "AI upskilling" programs often miss the mark because different skill levels require tailored strategies. Consider this simple example: training someone with basic AI literacy to become a technical expert requires different content than developing a technical expert into a deep specialist. Teaching advanced neural network architecture to someone who barely understands algorithms would be ineffective, while basic introductory courses would waste time for those ready to innovate.
To address this complexity, I've developed a three–tier classification system: AI literacy (basic understanding), technical expertise (implementation capabilities), and deep specialization (innovation capacity). This framework helps organizations develop targeted interventions that match their specific needs.
For policymakers, the implications are clear: successful AI strategies must simultaneously develop domestic educational pipelines while creating environments that attract and retain specialized global talent. For business leaders, this means developing internal pathways that strategically upskill talent based on specific organizational needs rather than pursuing generic development.
As specialized talent flows reshape along geopolitical lines, nations that implement data–informed, targeted talent development strategies will gain a competitive edge. Success depends on both nurturing domestic expertise and creating environments that attract global innovators, moving beyond generic approaches to address the specific needs of each AI ecosystem.