penAI’s GPT-3 is an artificial intelligence system that creates remarkably human-like text based on a prompt given by the user. Despite the impressive innovation behind the system, GPT-3 remains extremely biased in the way it autocompletes phrases, according to a new study by DisinfoLab, a student-led think tank at the College of William & Mary's Global Research Institute. A major contributor to this bias is the data that OpenAI used to train GPT-3. The system’s training data comes from a wide variety of sources––including Wikipedia and Reddit––which contain inherent biases that find themselves baked into GPT-3’s generations.
DisinfoLab’s report tested GPT-3’s bias in relation to one potential use for the system - its inclusion in search engines to autocomplete user’s search prompts. The study found that GPT-3 produced biased text predictions 43.83% of the time in a data set of 3,290 autocomplete predictions. Most of the algorithm’s negative bias pertained to phrases about sexuality and gender (57.98%). It showed lower rates of bias for predictions about race and religion (38.05%).
Every language generation model requires researchers to put the model’s algorithm through an intense training process. GPT-3 is no exception, though researchers were able to expedite its training process thanks to a supercomputer provided by Microsoft. Through this training, GPT-3 acquired 175 billion parameters, which are mathematical expressions that identify language patterns. This number is 10 times more than the last iteration of the model, GPT-2.
GPT-3 trained with data from five main sources, according to the programmers who developed the model. The choice of these sources of data and the selections of text within these sources remain undisclosed. Researchers have found biased text in several of the key sources that OpenAI used to train GPT-3, which creates bias in GPT-3’s text generations.
Training Data Sources and Bias
The largest contributor is the Common Crawl dataset, offering 410 billion tokens which hold 60% weight in the algorithm’s mix of training data. Tokens are a series of characters––including single words or word phrases––that GPT-3 uses as reference for its generations. The Common Crawl dataset is text collected by automated bots that scan web pages and index all texts, links, and metadata. Much of the common crawl is redundant. As such, OpenAI filtered out duplicate phrases to improve the quality of the dataset. However, OpenAI made no mention of steps taken to filter out biased or false content from the Common Crawl.
The second-largest contributor is WebText2, which contains text from webpages whose links appear in Reddit posts with three or more upvotes. Overall, WebText2 includes 45 million links from Reddit, resulting in 19 billion tokens with a weight of 22% in the training mix. It is further noteworthy that the majority of Reddit users are men, which affects the type of text collected. Researchers have discovered notable gender bias, religious bias, and ethnic bias in Reddit communities. Moreover, a threshold of three upvotes is small, meaning that OpenAI trained GPT-3 with fringe links that reached very small audiences.
The third and fourth datasets are two digital collections of books, Books1 and Books2. GPT-3 researchers do not elaborate how many or which books are in the dataset, or from when they were originally written. Books1 contributes 12 billion tokens, which hold an 8% weight in training mix; Books2 contributes 55 billion tokens, which also hold an 8% weight in training mix.
Finally, the smallest contributor of tokens to GPT-3 is Wikipedia with 3 billion tokens, which only have a 3% weight in the training mix. Wikipedia––much like the Common Crawl data or Reddit links––is loosely moderated, and therefore includes stereotypes and falsehoods given the ability of users to edit the text.
Safeguards Against Bias in Future AI Models
Three of the five sources used to train GPT-3 have direct links to bias, because they pull from unmoderated content without any deliberate actions to filter out false or misleading information. For the other two sources––Books1 and Books2––this pattern likely holds. Though we don’t know what specific digital books are part of these datasets, it is unlikely that OpenAI would safeguard against bias for Books1 and Books2 without doing the same for the other sources. At a minimum, the uncertainty of the extent of bias in these sources makes it more difficult to combat.
This discussion brings up a critical question; would a different selection of training sources eliminate bias in GPT-3? Not necessarily. Large training data sets like the ones utilized by GPT-3 contain human generated text, which is riddled with bias, errors, and inconsistencies. While we may not be able to eliminate bias, we can mitigate it. A productive first step for OpenAI would be to publish GPT-3’s training set so that researchers can analyze it for bias. If a certain source uniquely contributes to bias, OpenAI can reevaluate its inclusion in the algorithm by adjusting its weight or excluding it altogether.
OpenAI may also consider implementing stronger post-learning protections against bias, beyond their current banners that flag content which may contain harmful sentiment. For instance, they could develop active moderation algorithms that detect and eliminate bias. Moreover, OpenAI could add a feature that allows users to report biased generations. Currently, OpenAI only lets users judge if a generation is “useful” or “poor.”
OpenAI should treat the investigation of GPT-3’s bias as an urgent matter, not only because the company recently released the algorithm to the public, but also because it plans to develop and release another interaction of the model, GPT-4. This future model will have over 500 times the number of parameters of GPT-3, according to the CEO of Cerebras, a chip-building company that collaborates with OpenAI.
This next model’s complexity and sheer volume of data will present an even larger obstacle to future researchers attempting to detect and eliminate biases. Already, training GPT-3 was expensive, energy-intensive, and long-lasting. To retrain GPT-3 for bias mitigation would be costly. For GPT-4, the problem would be exponentially worse. Retraining its data set retroactively would demand extremely high amounts of capital, energy, and time. Therefore, OpenAI must take action to address the bias before training GPT-4. Plans for GPT-4 indicate that it won't be ready for several years, so researchers should prepare clear steps that OpenAI can follow to minimize biased sources before it develops and trains this next model.
a global affairs media network
AI Training Data Contributes To Its Bias
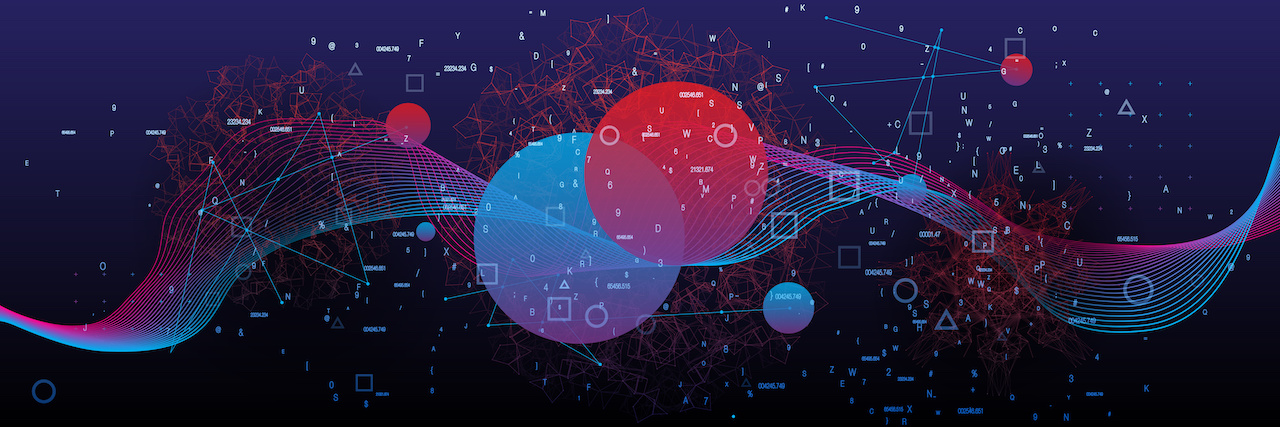
Image via Adobe Stock.
January 28, 2022
In an article explaining the ramifications of their report on identity bias in GPT-3 and Google Search, DisinfoLab's directors break down how the sources of training data that make GPT-3 such a complex and accomplished AI also embed bias deep within its DNA.
O
penAI’s GPT-3 is an artificial intelligence system that creates remarkably human-like text based on a prompt given by the user. Despite the impressive innovation behind the system, GPT-3 remains extremely biased in the way it autocompletes phrases, according to a new study by DisinfoLab, a student-led think tank at the College of William & Mary's Global Research Institute. A major contributor to this bias is the data that OpenAI used to train GPT-3. The system’s training data comes from a wide variety of sources––including Wikipedia and Reddit––which contain inherent biases that find themselves baked into GPT-3’s generations.
DisinfoLab’s report tested GPT-3’s bias in relation to one potential use for the system - its inclusion in search engines to autocomplete user’s search prompts. The study found that GPT-3 produced biased text predictions 43.83% of the time in a data set of 3,290 autocomplete predictions. Most of the algorithm’s negative bias pertained to phrases about sexuality and gender (57.98%). It showed lower rates of bias for predictions about race and religion (38.05%).
Every language generation model requires researchers to put the model’s algorithm through an intense training process. GPT-3 is no exception, though researchers were able to expedite its training process thanks to a supercomputer provided by Microsoft. Through this training, GPT-3 acquired 175 billion parameters, which are mathematical expressions that identify language patterns. This number is 10 times more than the last iteration of the model, GPT-2.
GPT-3 trained with data from five main sources, according to the programmers who developed the model. The choice of these sources of data and the selections of text within these sources remain undisclosed. Researchers have found biased text in several of the key sources that OpenAI used to train GPT-3, which creates bias in GPT-3’s text generations.
Training Data Sources and Bias
The largest contributor is the Common Crawl dataset, offering 410 billion tokens which hold 60% weight in the algorithm’s mix of training data. Tokens are a series of characters––including single words or word phrases––that GPT-3 uses as reference for its generations. The Common Crawl dataset is text collected by automated bots that scan web pages and index all texts, links, and metadata. Much of the common crawl is redundant. As such, OpenAI filtered out duplicate phrases to improve the quality of the dataset. However, OpenAI made no mention of steps taken to filter out biased or false content from the Common Crawl.
The second-largest contributor is WebText2, which contains text from webpages whose links appear in Reddit posts with three or more upvotes. Overall, WebText2 includes 45 million links from Reddit, resulting in 19 billion tokens with a weight of 22% in the training mix. It is further noteworthy that the majority of Reddit users are men, which affects the type of text collected. Researchers have discovered notable gender bias, religious bias, and ethnic bias in Reddit communities. Moreover, a threshold of three upvotes is small, meaning that OpenAI trained GPT-3 with fringe links that reached very small audiences.
The third and fourth datasets are two digital collections of books, Books1 and Books2. GPT-3 researchers do not elaborate how many or which books are in the dataset, or from when they were originally written. Books1 contributes 12 billion tokens, which hold an 8% weight in training mix; Books2 contributes 55 billion tokens, which also hold an 8% weight in training mix.
Finally, the smallest contributor of tokens to GPT-3 is Wikipedia with 3 billion tokens, which only have a 3% weight in the training mix. Wikipedia––much like the Common Crawl data or Reddit links––is loosely moderated, and therefore includes stereotypes and falsehoods given the ability of users to edit the text.
Safeguards Against Bias in Future AI Models
Three of the five sources used to train GPT-3 have direct links to bias, because they pull from unmoderated content without any deliberate actions to filter out false or misleading information. For the other two sources––Books1 and Books2––this pattern likely holds. Though we don’t know what specific digital books are part of these datasets, it is unlikely that OpenAI would safeguard against bias for Books1 and Books2 without doing the same for the other sources. At a minimum, the uncertainty of the extent of bias in these sources makes it more difficult to combat.
This discussion brings up a critical question; would a different selection of training sources eliminate bias in GPT-3? Not necessarily. Large training data sets like the ones utilized by GPT-3 contain human generated text, which is riddled with bias, errors, and inconsistencies. While we may not be able to eliminate bias, we can mitigate it. A productive first step for OpenAI would be to publish GPT-3’s training set so that researchers can analyze it for bias. If a certain source uniquely contributes to bias, OpenAI can reevaluate its inclusion in the algorithm by adjusting its weight or excluding it altogether.
OpenAI may also consider implementing stronger post-learning protections against bias, beyond their current banners that flag content which may contain harmful sentiment. For instance, they could develop active moderation algorithms that detect and eliminate bias. Moreover, OpenAI could add a feature that allows users to report biased generations. Currently, OpenAI only lets users judge if a generation is “useful” or “poor.”
OpenAI should treat the investigation of GPT-3’s bias as an urgent matter, not only because the company recently released the algorithm to the public, but also because it plans to develop and release another interaction of the model, GPT-4. This future model will have over 500 times the number of parameters of GPT-3, according to the CEO of Cerebras, a chip-building company that collaborates with OpenAI.
This next model’s complexity and sheer volume of data will present an even larger obstacle to future researchers attempting to detect and eliminate biases. Already, training GPT-3 was expensive, energy-intensive, and long-lasting. To retrain GPT-3 for bias mitigation would be costly. For GPT-4, the problem would be exponentially worse. Retraining its data set retroactively would demand extremely high amounts of capital, energy, and time. Therefore, OpenAI must take action to address the bias before training GPT-4. Plans for GPT-4 indicate that it won't be ready for several years, so researchers should prepare clear steps that OpenAI can follow to minimize biased sources before it develops and trains this next model.